3D Object Detection and Vehicle Classification based on LiDAR Point Clouds for Real-time Traffic Flow Monitoring
Research Team
Lead Researchers:
- Xunfei Jiang, Computer Science
- Bingbing Li, Manufacturing Systems and Engineering
- Xudong Jia, Civil Engineering
Collaborators:
Student Team:
-
Chen Li*, M.S. student in Computer Science at Columbia University
-
Tongzi (Peter) Wu, Research Assistant at ARCS
-
Alexander Rose*, M.S. student in Software Engineering at CSUN
-
Ruitao Wu, B.S. student in Computer Science at CSUN (ARCS Fellow)
-
Icess Nisce*, B.S. student in Computer Science at CSUN (ARCS Fellow)
-
Brian Uribe*, B.S. student in Computer Science at CSUN
-
Javier Carranza*, B.S. student in Computer Science at CSUN
-
Alondra Gonzalez*, B.S. student in Computer Science at CSUN
-
Matthew Davis*, B.S. student in Computer Science at CSUN
-
Andres Mercado*, B.S. student in Computer Science at CSUN
-
Ziaur Chowdhury, B.S. student in Computer Science at CSUN
-
Gustavo Velasquez Sanchez, B.S. in Computer Science at CSUN
-
Xin Gao, B.S. in Computer Science at CSUN
-
Cesar Villa, B.S. in Computer Science at CSUN
Funding
- Funding Organization:
- Funding Program:
Abstract
Vehicle detection plays an important role in analyzing traffic flow data for efficient planning in intelligent transportation. Machine Learning technology has been increasingly used for vehicle detection in both 2D real-time traffic flow video and 3D point clouds. Adverse weather conditions, such as fog, rain, snow, extreme wind, and other conditions prove to be challenging for 2D vehicle detection. 3D LiDAR point clouds can be more resistant to adverse weather conditions. Most of the existing research on 3D vehicle detection is used for autonomous vehicle driving with the LiDAR cameras deployed on vehicles. There is a lack of research on real-time vehicle detection for intelligent transportation with LiDAR cameras deployed by highway/freeway. In this project, we propose to build a system that collects real-time traffic flow data through 3D LiDAR cameras, processes the 3D point cloud data for vehicle detection and classification, and provides a web-based service with vehicles detected and classified in real-time traffic flow and data visualization for statistical traffic flow data.
Motivation/Research Problem
Object detection includes the parametrization of a bounding box containing the recognized and classified object. Most research in that area has focused on two-dimensional (2D) object detection based on widely available Red-Green-Blue (RGB) or grayscale images. However, this completely leaves out the third dimension and only partly imitates the human visual system. In fact, many modern applications, like robot assistants or autonomous vehicles, are highly dependent on depth and surface data to securely navigate in three-dimensional (3D) environments through LiDAR cameras. With the rising availability of mobile 3D sensors like RGB-Depth (RGB-D) cameras and LiDAR (light detection and ranging) sensors, more depth data can be captured and processed. This allowed great progress in the field of 3D object detection (3DOD) and brought forth a variety of heterogeneous methods and solutions used for this task.
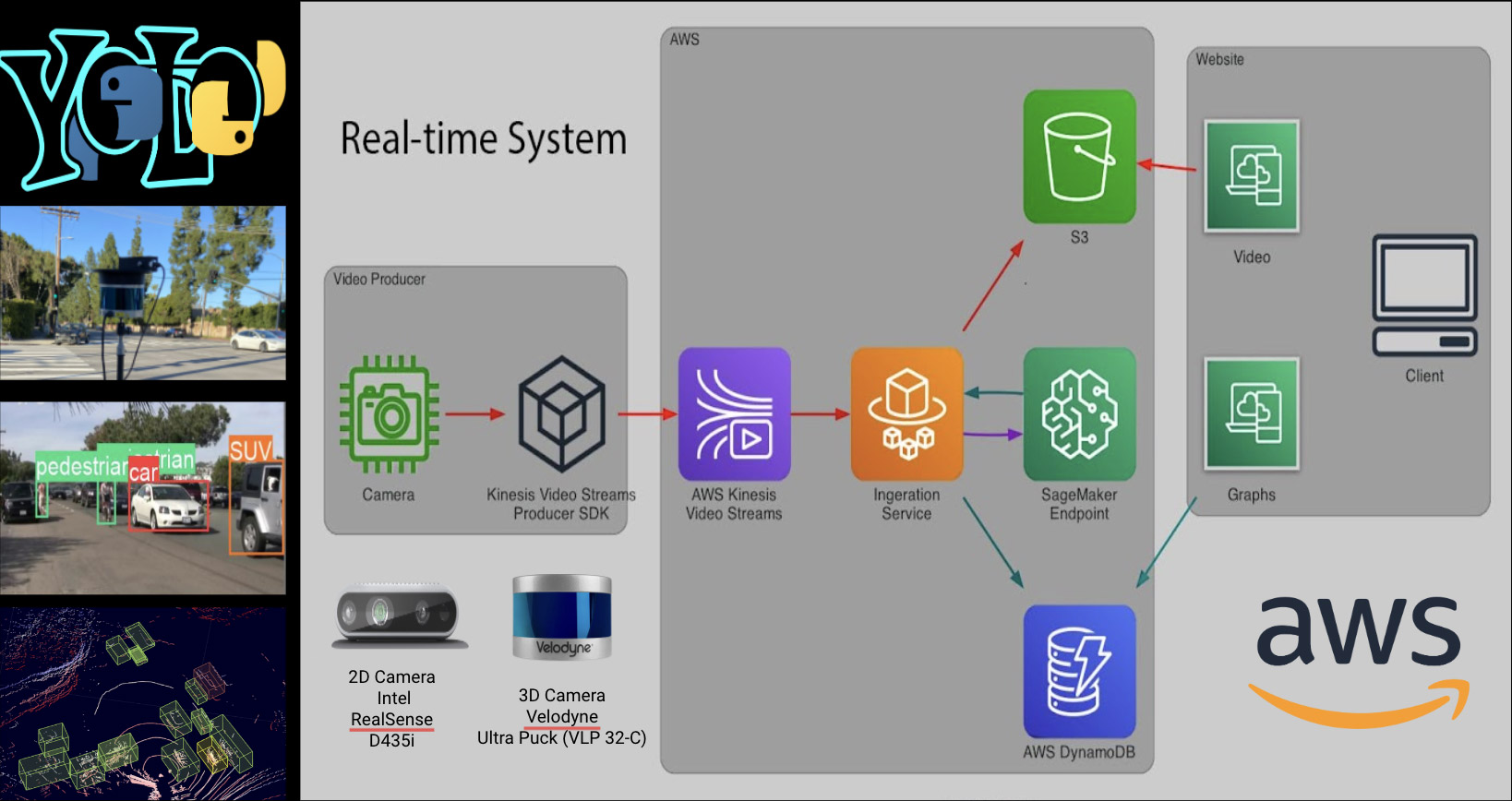
Research Questions and Research Objectives
The goal of this project is to detect and classify vehicle types and monitor the real-time traffic flow of highways in Southern California.
- Use Velodyne cameras to collect 3D LiDAR point cloud data for traffic flow on highways
- Automate the data calibration for matrices setting of 3D LiDAR camera
- Build 3D machine learning models to detect vehicles and classify vehicle types in 3D LiDAR point cloud data
- Develop a system that monitors and visualizes the real-time traffic flow of highways in South California
- Apply 3D vehicle detection and classification models on real-time LiDAR point cloud traffic flow and output vehicle detection and classification results, including vehicle types and coordinates, etc.
- Process the real-time vehicle detection results and generate statistical data for traffic flow, including number of vehicles in each type, traffic flow speed, density, etc.
- Visualize the real-time statistical vehicle detection and classification results and predict the traffic flow on highways
Research Methods
- Data Acquisition for Live Traffic Stream
- Live video is streamed from a camera connected edge device using Amazon Kinesis Video Streams, an AWS service that enables video ingestion to the cloud from mobile, IoT, and camera devices.
- Fragments from this video stream are consumed in real-time by an application server running on AWS Fargate, a serverless compute platform for containerized applications. These video fragments are deconstructed into individual frames and object detection is performed on each frame using a custom YOLO model deployed on SageMaker hosting services.
- SageMaker is an AWS suite of services that supports various ML solutions, including real-time serverless inference. Video frames are processed by the object detection application in parallel. Bounding boxes are then drawn on processed video frames, which are then reconstructed into video fragments and saved to Amazon S3, an AWS storage solution.
- The detection metrics are recorded in Amazon DynamoDB, a NoSQL database service managed by AWS.
- Background Filtering for 3D Machine Learning Model
- Isolates dynamic targets by filtering out static features, such as buildings, from LiDAR data.
- Reduces the computational burden for 3D detection models.
- Mitigates the latency challenges posed by resource-intensive models.
- ComplexYOLO for 3D Vehicle Detection and Classification
- Performs object detection and classification using Birds-Eye-View projections of LiDAR point clouds.
- Utilizes a modified YOLOv2 architecture for real-time processing.
- Adds an Euler-Region-Proposal Network (E-RPN) to estimate object orientations.
- 2D Machine Learning Model
- The YOLOv8 model was chosen for its real-time, end to end inference.
- It was trained on our 2D roadside image dataset.
Research Deliverables and Products
- A real-time system that presents both 3D traffic flow with vehicles detected and labeled in the traffic streams
- 3D vehicle detection and classification models
- Conference and Journal papers in the areas of Intelligent Transportation and Machine Learning for Vehicle Detection
- Publications:
- A. Rose, I. Nisce, A. Gonzalez, M. Davis, B. Uribe, J. Carranza, J. Flores, X.-D. Jia, B.-B. Li, X.-F. Jiang, “A Cloud-based Real-Time Traffic Monitoring System with Lidar-based Vehicle Detection,” 2023 IEEE Green Energy and Smart Systems Conference (IGESSC), Long Beach, CA, USA, 2023, pp. 1-6, doi: 10.1109/IGESSC59090.2023.10321763.
- R. Wu, Z. Chowdhury, G. V. Sanchez, X. Gao, C. Villa and X. Jiang, “Real-time Vehicle Detection System for Intelligent Transportation using Machine Learning,” 2022 IEEE Green Energy and Smart System Systems (IGESSC), Long Beach, CA, USA, 2022, pp. 1-6, doi: 10.1109/IGESSC55810.2022.9955329.
Research Timeline
Start Date: 02/01/2021
End Date: TBD
Lead Researchers:
- Xunfei Jiang, Computer Science
- Bingbing Li, Manufacturing Systems and Engineering
- Xudong Jia, Civil Engineering
Collaborators:
Student Team:
- Johnathan Cordova*, MS in Computer Science (ARCS Fellow)
- Rachel Gilyard*, MS in Computer Science
- Robin Rosculete*, B.S. in Computer Science
- Jose Flores*, B.S. in Computer Science
- Jimwell Castillo*, Gisela Calva*, Anthony Merzoian*, Raymond Gharapeti Babayans*, Alma Dollente*, Kaylee Groves* (Computer Science Senior Design 2023-2024 Team)
- Param Ridham Desai*, B.S. in Computer Science
- Mahi Kachhadiya*, B.S. in Computer Science
- Nabiha Sharif* (Taft High School STEAM Magnet)
- Chen Li, M.S. student in Computer Science at Columbia University
- Tongzi (Peter) Wu, Research Assistant at ARCS
- Alexander Rose, M.S. in Software Engineering (ARCS Fellow)
- Ruitao Wu, B.S. student in Computer Science (ARCS Fellow)
- Icess Nisce, B.S. student in Computer Science (ARCS Fellow)
- Brian Uribe, Javier Carranza, Alondra Gonzalez, Matthew Davis (Computer Science Senior Design 2022-2023 Team)
- Andres Mercado, B.S. student in Computer Science
- Ziaur Chowdhury, Gustavo Velasquez Sanchez, Xin Gao, Cesar Villa (Computer Science Senior Design 2021-2022 Team)
Note: names marked with an asterisk (*) indicate current students
Funding
- Funding Organization:
- Funding Program: