Research Project
Astro Cultivators: Autonomous Growth System for Space Farming
(Astro Cultivator Deployment for Freight Farming)
Research Team
Lead Researchers:
- Dr. Bingbing Li, Manufacturing Systems Engineering
- Dr. Xunfei Jiang, Computer Science
- Dr. Annette Besnilian, Director, Marilyn Magaram Center for Food Science, Nutrition and Dietetics (MMC)
- Andreas George, Project Coordinator (Garden), MMC
Collaborators:
- April Diederich, Andreas George, Julie Miller, Marilyn Magaram Center for Food Science, Nutrition, and Dietetics
- CSUN Institute for Sustainability
- LAPD PALS
- Ralph Fritsche, NASA Kennedy Space Center
Student Team:
- Anthony Morales-Badajoz, graduate student in Computer Engineering
- Neville Elieh, undergraduate student in Computer Science
- Elliot Sadler, graduate student in Manufacturing Systems Engineering
- Jasmine Glover, undergraduate student in Mechanical Engineering
- Manoj Nizampatnam, graduate student in Computer Science
- Andrew Wang, student at Portola High School
- Larry Zhang, student at Agoura High School
- Troy Israel, MS, Computer Engineering
- John Vega, MS, Software Engineering
- Jade Dergevorkian, BS, Comp Science
- Aram Boyrazian, BS, Comp Science
- Diego Carbajal, BS, Comp Science
- Arin Deravanesian, BS, Comp Science
- Mario Choto Guerrero BS, Comp Science
- Nik Khandandel, MS, Manufacturing Systems Engineering
Funding
- Funding Organization: Donation from CSUN Alumni Mrs. Susan Ettinger
- Funding Program: NASA Deep Space Food Challenge Phase 1 and Phase 2
SYNOPSIS
- Autonomous Robotic System for Crop Harvesting. The system leverages a robotic arm that is integrated with depth cameras, temperature sensors, and Computer-Aided Designed (CAD) end effectors to autonomously harvest crops inside a freight farm container.
- A hyperspectral camera captures detailed spectral data to assess plant health. The data is processed through a 3D Convolutional Neural Network (CNN) machine learning model, which classifies crops as healthy or unhealthy based on spectral patterns.
- The system employs depth cameras, temperature sensors and other environmental monitoring tools to enable precise real-time navigation and operation of the robotic arm. The sensors and machine learning models allow the robotic arm to navigate its surroundings and harvest crops with minimal human intervention.
Abstract
The autonomous space farming system could be used to grow self-pollinating crops in space exploration missions that require no human intervention. Our goal is to utilize hyperspectral imaging and traditional imaging to allow for machine learning models to be used and retrained for not only our crops, but others as well. Temperature, humidity, and pressure sensors coupled with a robotic arm will care for and tend to the crops at every stage of development. This system can decrease crew members’ input needed to operate the plant growth systems in deep space by providing autonomous monitoring, real-time data reporting, ambient environment management, automatic harvesting, and cleaning. Crops with high nutrient content, high acceptability ratings, dwarf growth habits, and short harvest cycles (Sugar Ann Snap Peas and Red Robin Tomato) will initially be grown in this modular system, which has the potential to be scaled up or down depending on the mission. A variety of “pick and eat” crops can be grown to provide palatable, safe, nutritious foods that are familiar foods for crews to consume. To reduce resupply from Earth, the seeds from these crops have the potential to be saved and used to grow continuous generations of crops.
Motivation/Research Problem
The major mission of space food development is to provide a safe, nutritious, and acceptable food system that can function for a long-duration spaceflight, such as a human Mars mission in the 2030s. Researchers have gained large progress on plant growth in space stations in the past 40 years; however, NASA’s Advanced Food Technology Project (AFTP) ISS food system is inadequate for 5-year missions beyond Low Earth Orbit (LEO) (i.e. storage). The two main causes of long-term storage (i.e. longer than 3 years) were nutritional content of the food and inadequate food acceptability, which led to insufficient intake by the crew. Missions to Mars will rely on packaged foods from Earth, which will meet the macronutrient needs of astronauts but are subject to degradation of vitamin C, vitamin B1 and are deficient in vitamin K. Providing familiar foods from Earth and growing fresh produce to supplement nutrient deficiencies will be vital for the health of the crew. Space food planting is still mainly managed by the crew, which has brought up challenges for crew who are not scientists in agriculture. There is an urgent need for an autonomous system to manage the growth of plants from seed to seed.
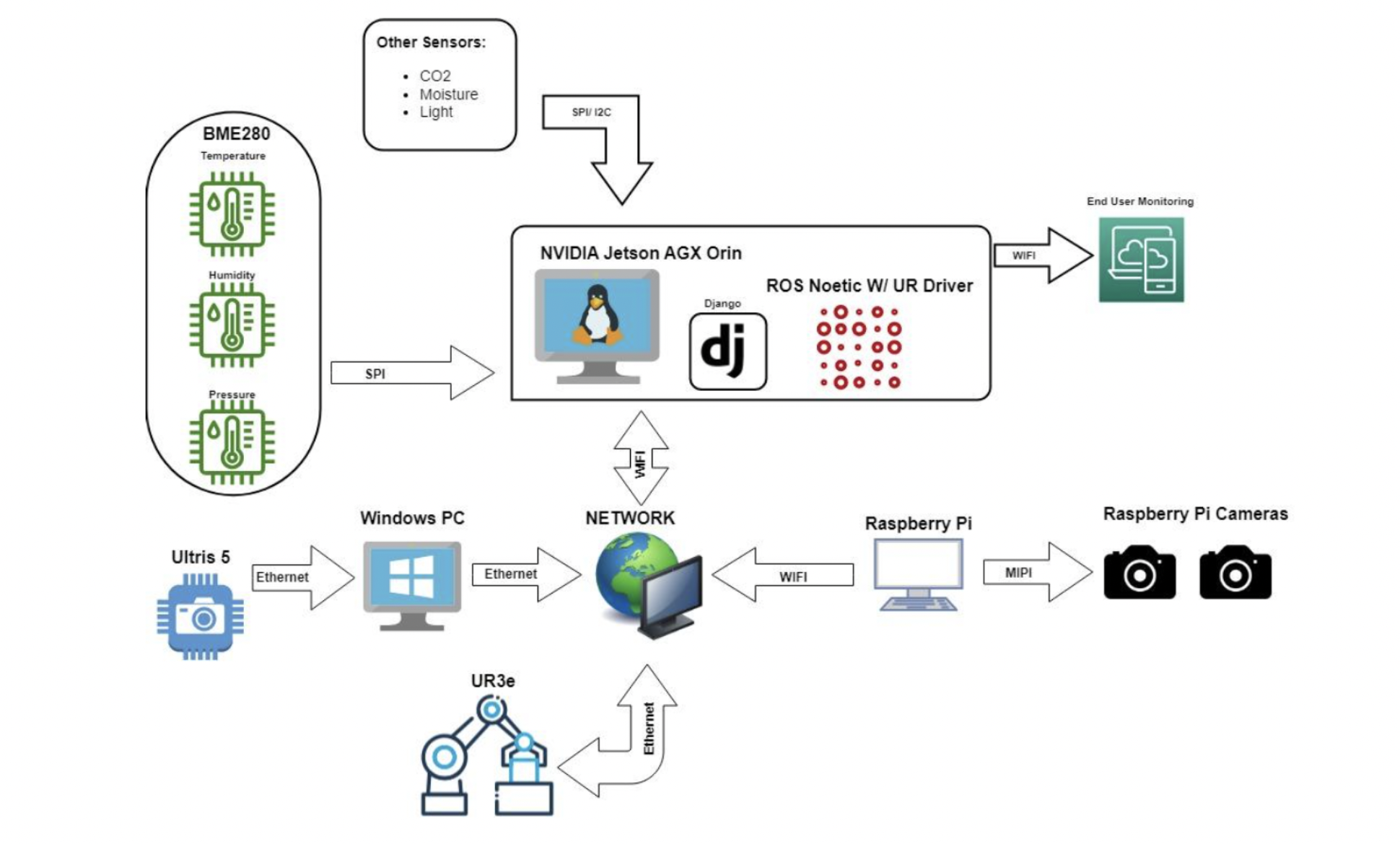
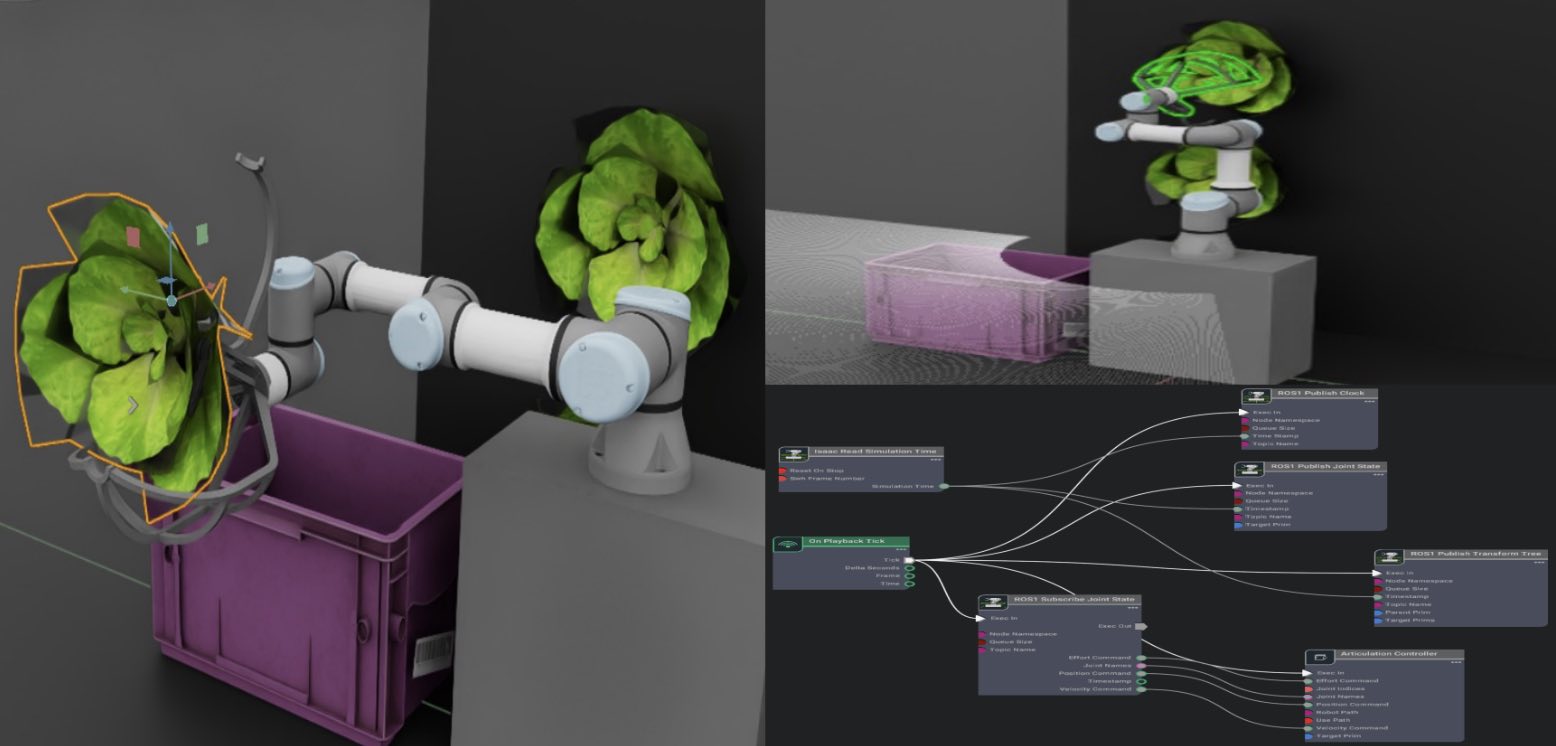
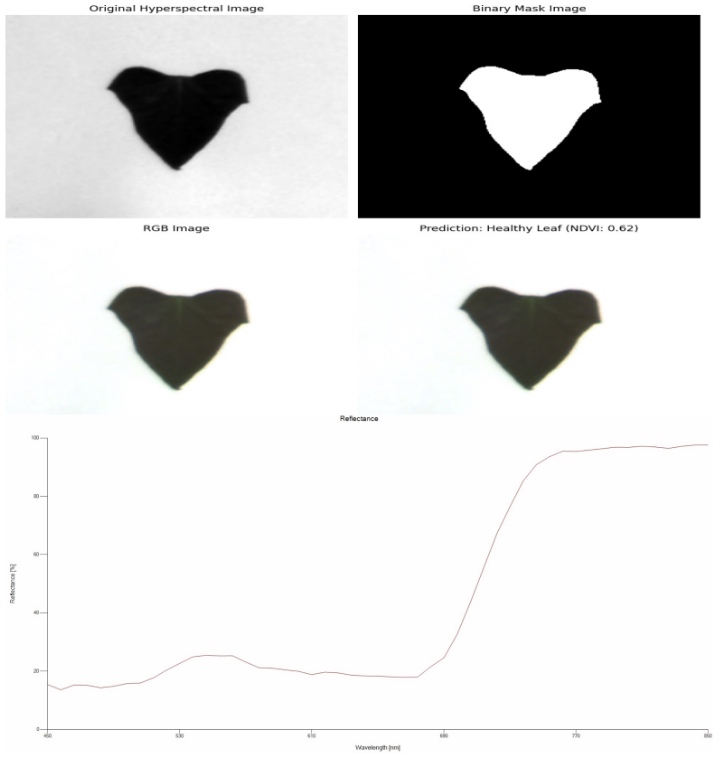
Research Questions and Research Objectives
In this project, we aim to build an autonomous space farming system that could be utilized in space exploration missions without human intervention. The system will provide autonomous monitoring, real-time data reporting, ambient environment management, and automatic harvesting and cleaning. It will be developed to meet these favorable qualities through Artificial Intelligence (AI) and Controlled Environment Agriculture (CEA).
- Optimize agricultural techniques for growing nutrient-rich produce essential for maintaining the health and well-being of astronauts during extended space missions. The system will also address terrestrial agricultural challenges by adapting the autonomous system to controlled environments such as vertical farms and urban agricultural settings.
- Implement and train machine learning models that facilitate autonomous crop monitoring and plant health assessment. These models will enable the system to operate independently, making real-time decisions based on image classification, object detection, and plant health for both space and terrestrial applications.
- Train deep learning model responsible for trajectory and path prediction of the robotic arm throughout the harvesting motion. This model will account for and train based on positioning of a RealSense depth camera and LiDAR sensor with reference to the robotic arm and end effector.
Research Methods
-
Test and verify the accuracy of Object Detection and Image Classification model performance based on the hyperspectral and RGB data. RGB data is used to detect the crops, and the hyperspectral data is used for plant health diagnostics.
-
Execute harvesting motions utilizing the joint trajectory controller while recording simulated LiDAR sensor, depth camera, and RGB camera to have strong examples of desired behavior to guide deep learning agent.
The food technology is to be housed by an enclosure containing an adept automated plant health monitoring system equipped with imaging sensors and passive sensors, all of which be used in conducting non-destructive analysis. This system will be used to produce crops, such as tomatoes, using NASA plant bags that are filled with a medium consistent with Martian soil. A variety of “pick and eat” crops will be grown to provide palatable, safe, nutritious foods that are familiar foods for crews to consume. To reduce resupply from Earth, the seeds from these crops should have the potential to be saved and used to grow continuous generations of crops. The space farming system could manage the environmental elements and support operations for data transmission from input devices using a main control module, and provide real-time assessment of the environment and plant health conditions. This includes assessments of roots, stems, and leaves. The automatic computing and analysis results in an adaptive system that reduces human intervention while promoting plant growth and health, as well as real-time visualization.
Research Deliverables and Products
- Hardware Components: NVIDIA Jetson AGX Orin Development Kit, UR3e
Robotic Arm, Cubert S5 Hyperspectral Camera, Intel RealSense Depth
Camera, LiDAR sensor, temperature sensor, humidity sensor and RGB
camera. - Software Components: Robot Operating System (ROS), NVIDIA Omniverse
(IssacSim), Nvidia JetPack SDK, Python, PyTorch, OpenCV, YOLOV7, Flask,
Apache Web Server.
- One ACM conference paper accepted for publication: Anthony Morales-Badajoz, Neville Elieh, April Diederich, Elliot Sadler, Jasmine Glover, Manoj Nizampatnam, Troy Israel, Andrew Wang, Larry Zhang, Annette Besnilian, Andreas George, Julie Miller, Xunfei Jiang, Bingbing Li*, “Astro Cultivators: Autonomous Growth System for Space Farming based on Machine Vision and Multi-Sensor Fusion”, Proceedings of Cyber-Physical Systems and Internet of Things Week 2023 (CPS-IoT Week Workshops ’23), San Antonio, Texas USA, May 9-12, 2023.
- An autonomous farming system that monitors the growth of plants, controls a robot arm to perform cleaning and harvesting.
- Intelligent algorithms to detect the stems, leaves and fruits, and classify the growth and health condition of the plants.
- Conference and Journal papers in the areas of smart agriculture, cyber-physical system (CPS), and Internet of things (IoT).
Commercialization Opportunities
- Application: Food plants monitoring system
- Key Values: Home, school, and community garden/nutrition monitoring system that is low-cost and controlled with a mobile phone in real-time.
- Potential Customers: Home gardeners, schools, community gardens, food desert residents.
Research Timeline
Start Date: 02/01/2021
End Date: TBD
Lead Researchers:
- Dr. Bingbing Li, Manufacturing Systems Engineering
- Dr. Xunfei Jiang, Computer Science
- Dr. Annette Besnilian, Director, Marilyn Magaram Center for Food Science, Nutrition and Dietetics (MMC)
- Andreas George, Project Coordinator (Garden), MMC
Collaborators:
- April Diederich, Andreas George, Julie Miller, Marilyn Magaram Center for Food Science, Nutrition, and Dietetics
- CSUN Institute for Sustainability
- LAPD PALS
- Ralph Fritsche, NASA Kennedy Space Center
Student Team:
- Anthony Morales-Badajoz, graduate student in Computer Engineering
- Neville Elieh, undergraduate student in Computer Science
- Elliot Sadler, graduate student in Manufacturing Systems Engineering
- Jasmine Glover, undergraduate student in Mechanical Engineering
- Manoj Nizampatnam, graduate student in Computer Science
- Andrew Wang, student at Portola High School
- Larry Zhang, student at Agoura High School
- Troy Israel, MS, Computer Engineering
- John Vega, MS, Software Engineering
- Jade Dergevorkian, BS, Comp Science
- Aram Boyrazian, BS, Comp Science
- Diego Carbajal, BS, Comp Science
- Arin Deravanesian, BS, Comp Science
- Mario Choto Guerrero BS, Comp Science
- Nik Khandandel, MS, Manufacturing Systems Engineering
Funding
- Funding Organization: Donation from CSUN Alumni Mrs. Susan Ettinger
- Funding Program: NASA Deep Space Food Challenge Phase 1 and Phase 2