Research Project
Building Trust in Human-Machine Teams (HMT)
(Modeling Trust in Heterogeneous R3 Human-Machine Teams)
Lead Researchers
- Kevin Zemlicka, Academic Advisement, Anthropology & Psychology
- Nhut Ho – ARCS Director – Mechanical Engineering
Collaborators
- Ben Morrell
- Marcel Kaufmann
- Mike Milano, JPL
- Olivier Toupet, Jet Propulsion Laboratory
- Nelson Brown, NASA Armstrong Flight Research Facility
- Ali Agha, Jet Propulsion Laboratory
Student Team
- Zulma Lopez*
- Jordan Jannone*
- Rachel Huerta*
- Aniket Christi*
- Julia Spencer, Tran Le
- Jessica Steiner
- Dana Bellinger
- Samuel Mercado
- Andranik Yelanyan*
- Shaun Cabusas*
- Karen Dominguez*
- Cesar Flores*
Note: names marked with an asterisk (*) indicate current students
Funding
- Funding Organization: Air Force Office of Scientific Research
- Funding Program:
SYNOPSIS
- Study explores trust development in human-machine interactions using ethnographic, qualitative methods in real-world settings.
- Research focuses on heterogeneous human-machine teams (HMT) with different roles, capabilities, collocation, and collaboration durations.
- We investigate how these diverse factors influence trust and team performance in practical, real-world scenarios.
Abstract
The current project has identified a gap in research studying trust evolution and calibration in R3 contexts, that is, in a heterogenous team of multiple real highly-autonomous machine teammates and real human teammates operating in situations with real consequences. To address this gap, the current 5-year study seeks to obtain foundational lessons and insights on how trust is calibrated and evolves over time, identify how technology and non-technology-related factors (e.g., organizational, cultural, personal) influence trust evolution, validate extant theoretical trust models against trust calibration and evolution of heterogeneous R3 human-machine teams (HMTs), generate hypotheses for trust evolution and calibration in heterogeneous R3 HMT contexts, and formulate research questions and identify pathways to translate the results of this basic research project to applied research. The field study will be designed in the form of two case studies, one for each heterogeneous R3 HMT: NASA JPL’s Mars 2020 HMT and NASA JPL’s Team CoSTAR subTerranean robotics HMT. The researchers will employ a set of complementary qualitative methods: case study/extended case study, iterative Rapid Assessment Procedures, and a grounded theory approach. Potential contributions to the DoD and Air Force’s missions include recommendations for building appropriate trust in heterogeneous R3 HMTs, basic research results transitioned to applied research for current Air Force Research Laboratory initiatives, and informing advanced concepts of operations that involve heterogeneous R3 HMTs and related advanced autonomy technologies envisioned in the DoD autonomy roadmap.
JPL’s trailer of Team CoSTAR’s practice run of the DARPA Subterranean Challenge.
Team CoSTAR explaining how their robots can autonomously navigate off-road extreme conditions.
There has been an increased interest in human machine team trust within the past few decades, though much of existing research consists of laboratory-based or simulation-based environments. While such studies are valuable in understanding solution approaches, there is a current lack of literature describing trust evolution and calibration in real R3 contexts (involving real heterogeneous human-machine teammates, real systems, and real consequences). R3 systems are a powerful context for trust research because they provide high levels of face validity and offer real-life richness from which both technology and non-technology factors that influence trust can be identified. Beyond the lack of trust literature in R3 contexts, another important limitation of previous HMT trust literature is that most studies have been confined to a single operator interacting with a single machine. However, existing and proposed HMT architectures take on multiple forms allowing for complex heterogeneous teaming, e.g. multi-robot technologies categorized into team agents, hierarchical agents, and mixed initiative systems. The current project seeks to fill this gap through the proposal of a 5-year study, employing qualitative methodologies to inductively explore heterogeneous R3 HMTs in unique, state of the art active NASA projects.
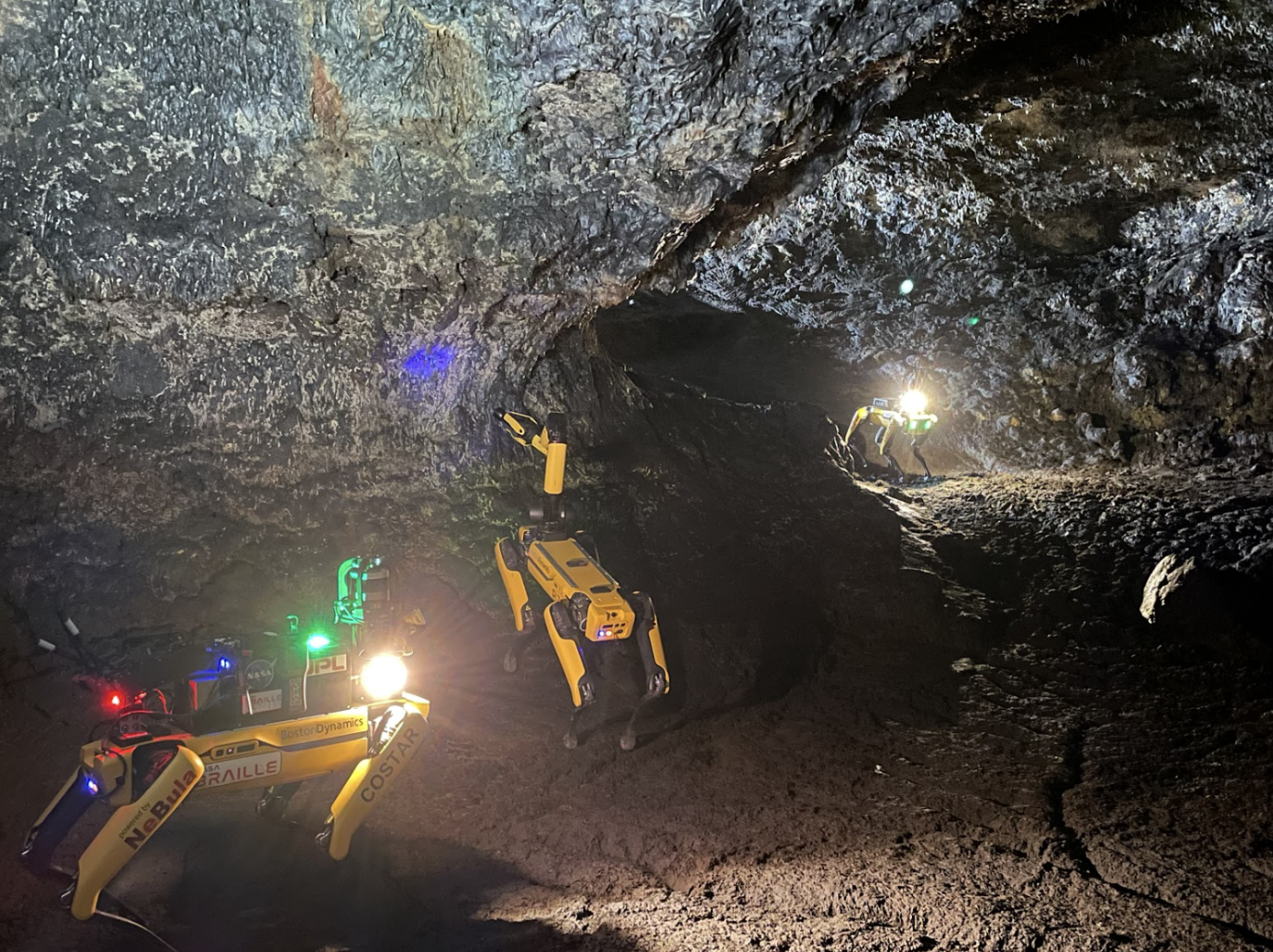
Team CoSTAR’s SPOT robots using their NeBULA software to explore a cavern.
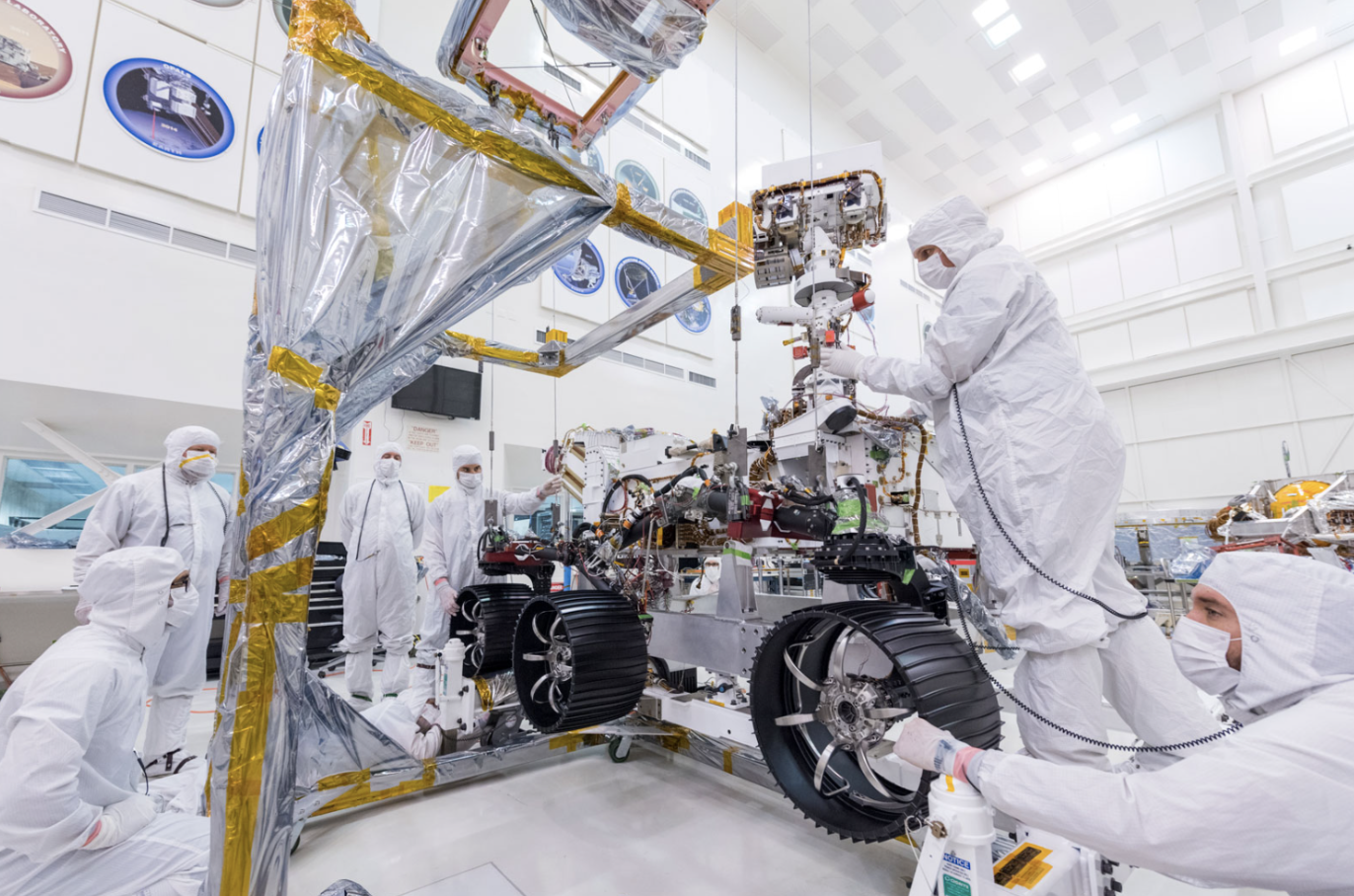
The Mars 2020 team building the Perseverance rover.
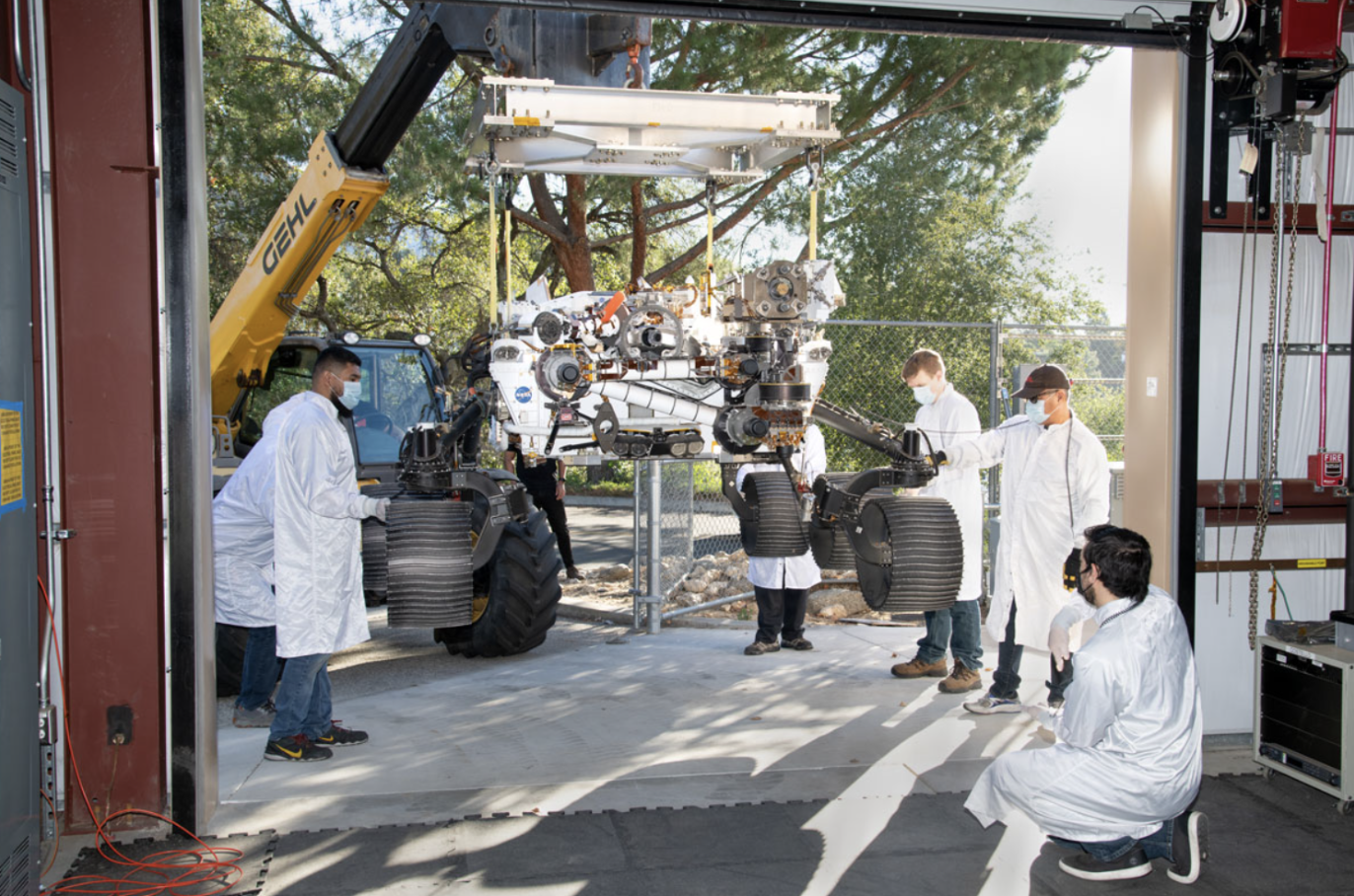
The Mars 2020 team building the Perseverance rover.
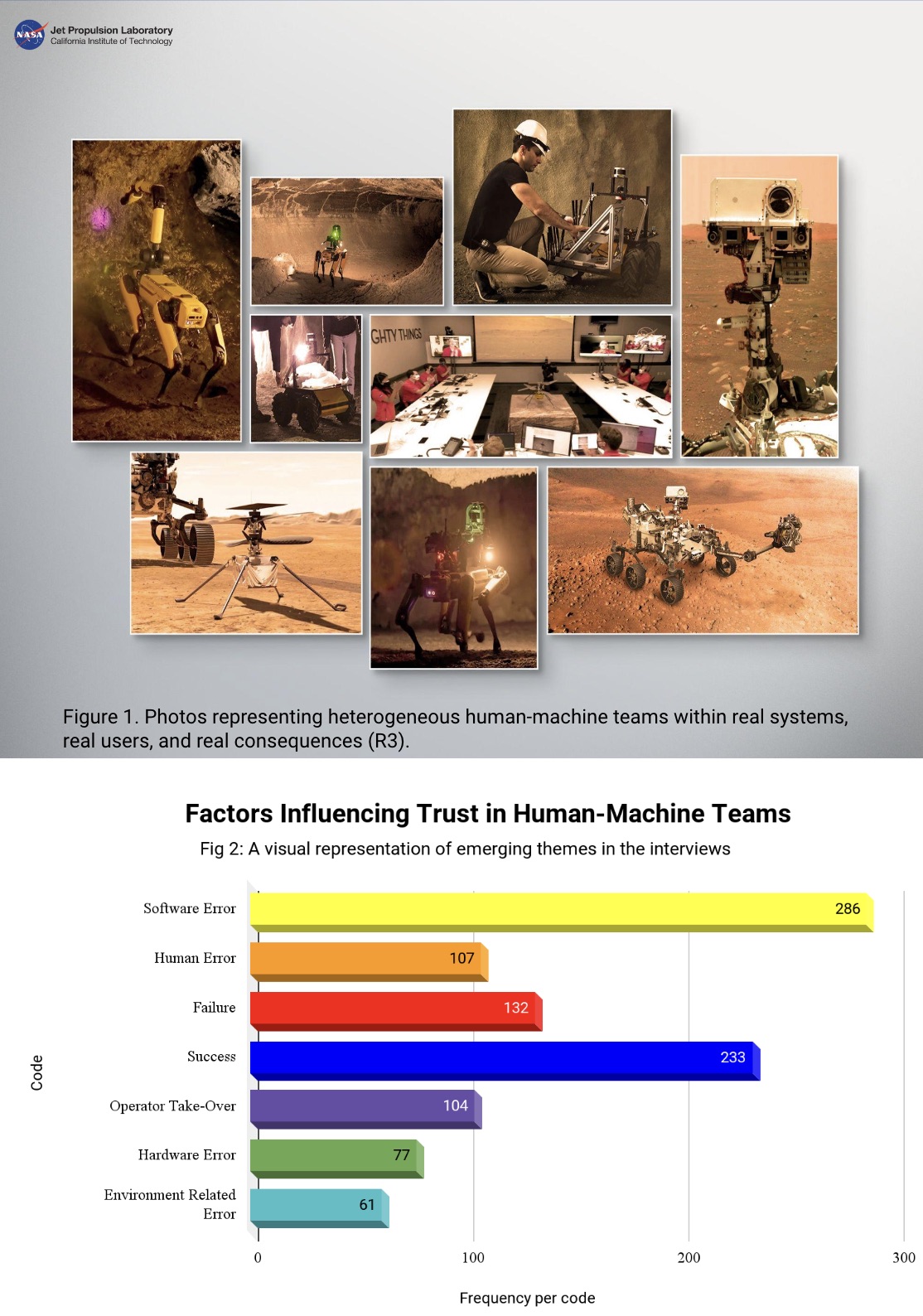
Alignment, Engagement and Contributions to the priorities of NASA’s Mission Directorates
Contributions include:
- Guidelines/recommendations for policy makers and designers of future Air Force systems to build appropriate trust for systems and concepts of operations that involve heterogeneous R3 HMTs with high levels of autonomy.
- Identify ways (via working with the AFOSR Trust and Influence program manager and colleagues at AFRL and DoD units involved in the three R3 HMTs) to transition research results to support applied research for current and important AFRL initiatives, such as Man-Unmanned Teaming (MuM-T), Concepts of Operations (ConOps), and Synthetic Teammate.
- Contribute to ConOps that involve heterogeneous HMTs and related advanced autonomy technologies (e.g., drone control algorithms, system health management, autonomy algorithms for experimental satellite systems) envisioned in the DoD’s autonomy roadmap.
Research Questions and Objectives
- Obtain foundational lessons and insights on how trust is calibrated and evolves over time.
- Identify how technology and non-technology-related factors (e.g., organizational, cultural, personal) influence the trust evolution.
- Validate extant theoretical trust models against trust calibration and evolution of heterogeneous Real World, Real Users, Real Consequences (R3) HMTs, and adapt or extend the models.
- Generate hypotheses for trust evolution and calibration in heterogeneous R3 HMT contexts.
Research Methods
- A case study method utilizing a multi-case design where technology and non-technology-related factors will be studied through interrelated cases for each heterogenous R3 HMT, in our case we will be focussing on the Mars Helicopter, NASA Armstrong’s Traveler, and JPL’s SubT team .
- An extended case method that applies a grounded theory approach to interview data, survey data, and participant observation data, in the analysis and revision of existing theories.
- An iterative Rapid Assessment Procedures method to give us insight into cultural values and meanings in a shorter timescale than one of a traditional ethnography. This method takes into account time constraints and limits imposed by Covid-19.
- An approach to validate and extend theoretical trust models that involves clarifying the operational definitions of the categories of factors that influence trust and verifying their relevance and comparing and contrasting existing data from literature review with emergent data across cases and three R3 HMTs.
Summary:
-
Use complementary qualitative methods for select R3 HMT at NASA JPL, including participant observation, surveys, and interviews (unstructured and semi-structured).
-
Analyze data using a grounded theory approach, involving thematic coding and a constant comparative method to generate hypotheses and new theoretical models.
-
Utilize an iterative case study method to refine design, preparation, and collection phases based on emergent themes or topics.
Research Deliverables and Products
-
Log data confirms our findings that knowledge of software is a high indicator of trust with R3 HMT.
-
Zoom meetings and ritualistic practices created a strong sense of communitas, enhancing trust and social cohesion without physical proximity.
-
Trust increases as humans become more grounded and take more ownership of robot behaviors/capabilities.
-
Higher stakes, complexity, and asset risk in Mars 2020 HMT reduced trust in robot team members, leading to more cautious use of autonomous features.
-
Hypothesis: The presence of a human safety operator in R3 HMT leads to more aggressive or risk-taking use of autonomous features.
Commercialization Opportunities
-
Develop a framework and guidelines to build trust in diverse Human-AI teams, emphasizing ethical AI design and responsible governance.
-
Inform advanced operations concepts involving heterogeneous HMTs and related advanced autonomy technologies by prioritizing people for responsible AI.
Research Timeline
Start Date: January 2021
End Date: January 2026
Lead Researchers
- Kevin Zemlicka, Academic Advisement, Anthropology & Psychology
- Nhut Ho – ARCS Director – Mechanical Engineering
Collaborators
- Ben Morrell
- Marcel Kaufmann
- Mike Milano, JPL
- Olivier Toupet, Jet Propulsion Laboratory
- Nelson Brown, NASA Armstrong Flight Research Facility
- Ali Agha, Jet Propulsion Laboratory
Student Team
- Zulma Lopez*
- Jordan Jannone*
- Rachel Huerta*
- Aniket Christi*
- Julia Spencer, Tran Le
- Jessica Steiner
- Dana Bellinger
- Samuel Mercado
- Andranik Yelanyan*
- Shaun Cabusas*
- Karen Dominguez*
- Cesar Flores*
Note: names marked with an asterisk (*) indicate current students
Funding
- Funding Organization: Air Force Office of Scientific Research
- Funding Program: