AI-assisted Knowledge Graph Design for the CADRE Robots
Lead Researchers:
- Bingbing Li, Manufacturing Systems Engineering
- Dr. Nhut Ho, Mechanical Engineering
Collaborators:
- Dr. Richard Otis, Technologist, JPL
- Dr. Thomas Lu, Technologist, JPL
- Diane Gault, Principle Experience Designer, Autodesk
- Daniele Grandi, System Engineer, Autodesk
- Daniel Noviello, Autodesk
- Vincenzo Ferrero, Mechanical Engineer, NIST
Student Team:
- Brandon Bian, Mathematics of Computation
- Max Slade, Anthropology
- Elliot Sadler, Mechanical Engineering
- Andrew Wang, High School Student
- Axel Fernandes, Engineering Management
- Bodia Borijin, Structural Engineering
Funding
- Funding Organization: Autodesk Inc, NASA
- Funding Program: Autodesk Research, MIRO
Abstract
Autodesk is investigating methods for improving the design of assemblies by learning best practices from previous designs, encoding this data in a knowledge graph, and assisting the designer during the detail design phases of the design process. Research focus is on the issues surrounding this transition from ideas into ordered graphs. Using a comprehensive collection of real-world CAD data as well as involving user research to identify what design knowledge to capture, a method to derive insights from the dataset is developed and the applicable insights used for a case study involving a redesign of the PUFFER/CADRE. Interviews with experienced technologists, designers, and engineers are performed to determine what design knowledge may provide support for specific systems they work on. A report outlining the findings will be submitted for publication. With this base in the transition from knowledge as an abstract into a programmable medium, such advancements as AI-assisted design or smart self-repair of remote machines are furthered.
Motivation/Research Problem
Autodesk is investigating methods for improving the design of assemblies by learning best practices from previous designs, encoding this data in a knowledge graph, and assisting the designer during the detail design phases of the design process.
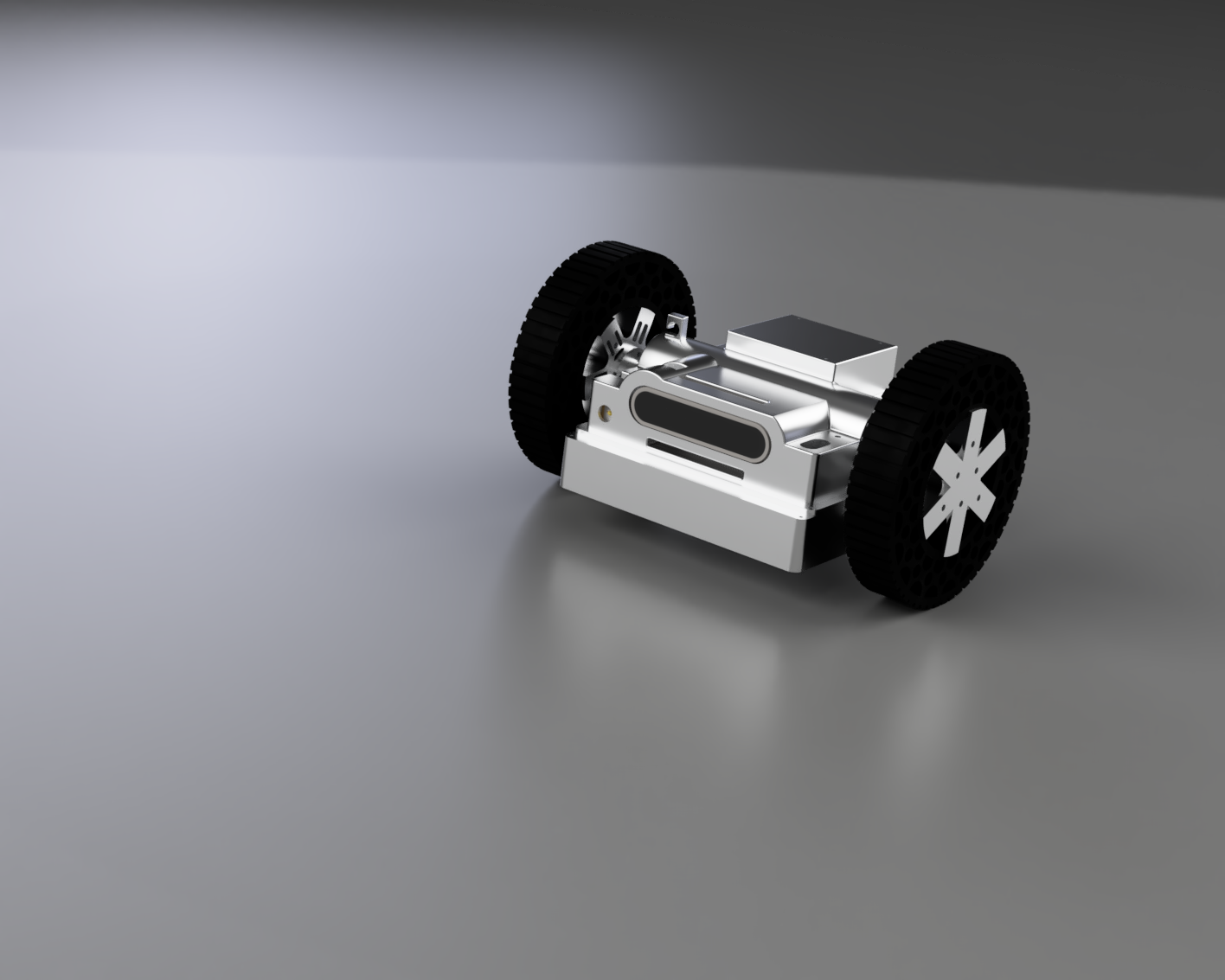
Alignment, Engagement and Contributions to the priorities of NASA’s Mission Directorates
With contribution and collaboration of representatives from JPL and Autodesk.
Research Questions and Research Objectives
Research focus is on the issues surrounding this transition from ideas into ordered graphs. Using a comprehensive collection of real-world CAD data as well as involving user research to identify what design knowledge to capture, a method to derive insights from the dataset is developed, and the applicable insights used for a case study involving a redesign of the PUFFER/CADRE.
Research Methods
Interviews with experienced technologists, designers, and engineers are performed to determine what design knowledge may provide support for specific systems they work on
Research Deliverables and Products
A report outlining the findings will be submitted for publication. With this base in the transition from knowledge as an abstract into a programmable medium, such advancements as AI-assisted design or smart self-repair of remote machines are furthered.
Research Timeline
Start Date: June 2021
End Date: May 2024
SLead Researchers:
- Bingbing Li, Manufacturing Systems Engineering
- Dr. Nhut Ho, Mechanical Engineering
Collaborators:
- Dr. Richard Otis, Technologist, JPL
- Dr. Thomas Lu, Technologist, JPL
- Diane Gault, Principle Experience Designer, Autodesk
- Daniele Grandi, System Engineer, Autodesk
- Daniel Noviello, Autodesk
- Vincenzo Ferrero, Mechanical Engineer, NIST
Student Team:
- Brandon Bian, Mathematics of Computation
- Max Slade, Anthropology
- Elliot Sadler, Mechanical Engineering
- Andrew Wang, High School Student
- Axel Fernandes, Engineering Management
- Bodia Borijin, Structural Engineering
Funding
- Funding Organization: Autodesk Inc, NASA
- Funding Program: Autodesk Research, MIRO