Research Project
Visualization of Hierarchical State Machines in a Multi-Agent Context
Abstract
Multi-agent autonomous systems with swarming behavior have been shown in laboratory studies and recent fielded demonstrations to provide a wide variety of benefits, including improved mission effectiveness, increased efficiency, cost savings, and safety (without direct human onboard). Despite these benefits, the complex behavior of these systems can be unpredictable, opaque, and counterintuitive, making it difficult for the operator to obtain proper situational awareness and to develop appropriate reliance, which ultimately may result in catastrophic accidents and loss of investment. Methods and approaches for creating interfaces to explain the complex behavior of these systems is an emergent field of study, and interfaces are often designed and developed through ad-hoc, evolutionary processes.
Motivation/Research Problem
- Develop a unified methodology to visualize behavior control, which is used to model and monitor activities in a multi-agent system.
- Assist users to know when there is a swarm in a behavior, what is happening to the system and what is happening with a particular agent.
- Connect local context and global context to help users identify an error and trace root cause(s) from unexpected behavior(s).
Research Team
Leading Researcher:
- Li Liu, Ph.D. Computer Science
Collaborator:
- So Young Kim-Castet, Group Supervisor – Human Systems Integration, JPL
- Scott Davidoff, Ph.D, JPL.
- Federico Rossi, Ph.D, JPL.
Student Team:
- Gage Aschenbrenner
- Ramin Roufeh
Funding
- Funding Organization: NASA
- Funding Program: MIRO
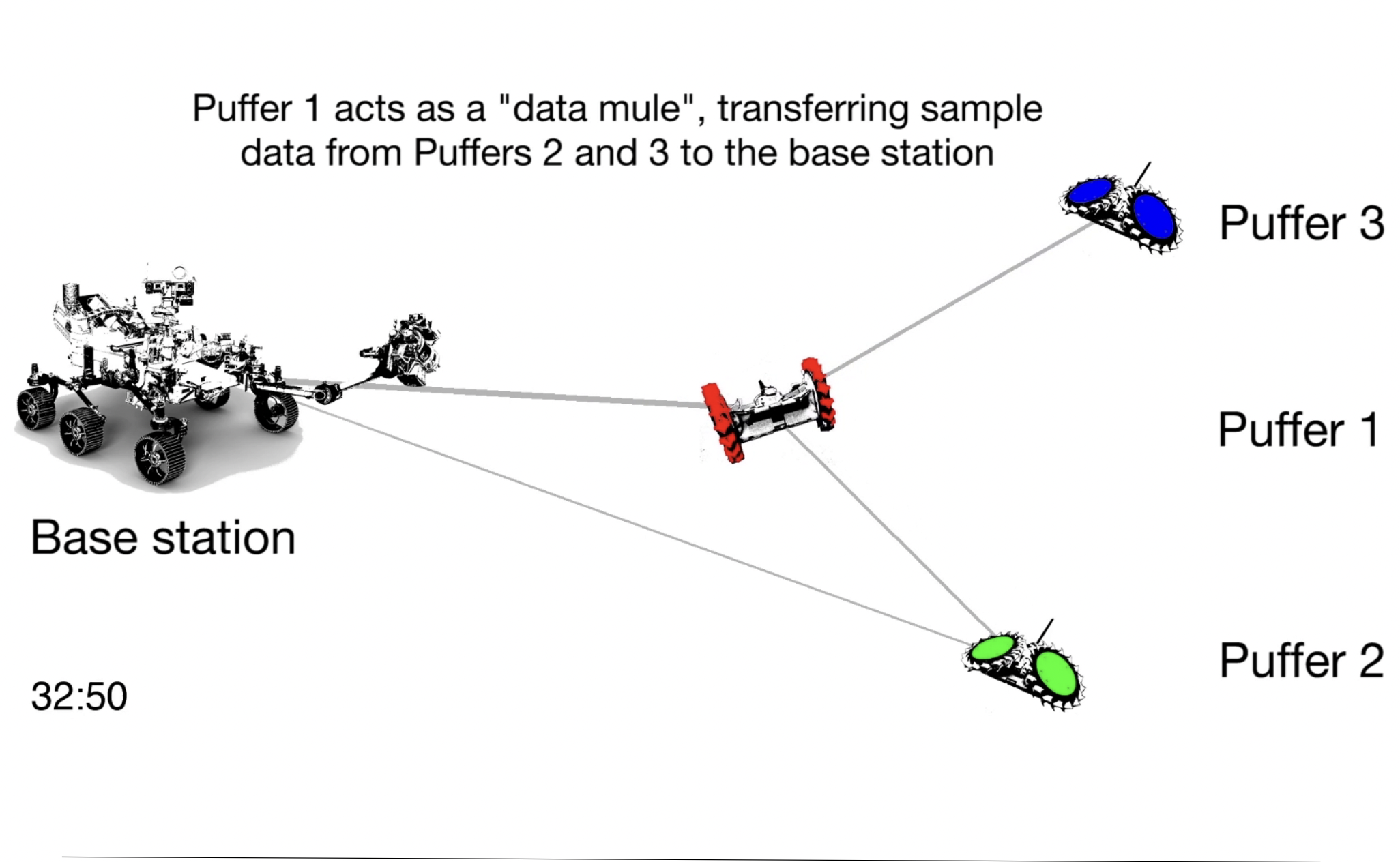
Research Team
Leading Researcher:
- Li Liu, Ph.D. Computer Science
Collaborator:
- So Young Kim-Castet, Group Supervisor – Human Systems Integration, JPL
- Scott Davidoff, Ph.D, JPL.
- Federico Rossi, Ph.D, JPL.
Student Team:
- Gage Aschenbrenner
- Ramin Roufeh
Funding
- Funding Organization: NASA
- Funding Program: MIRO